Network Models of COVID-19
March, 2020
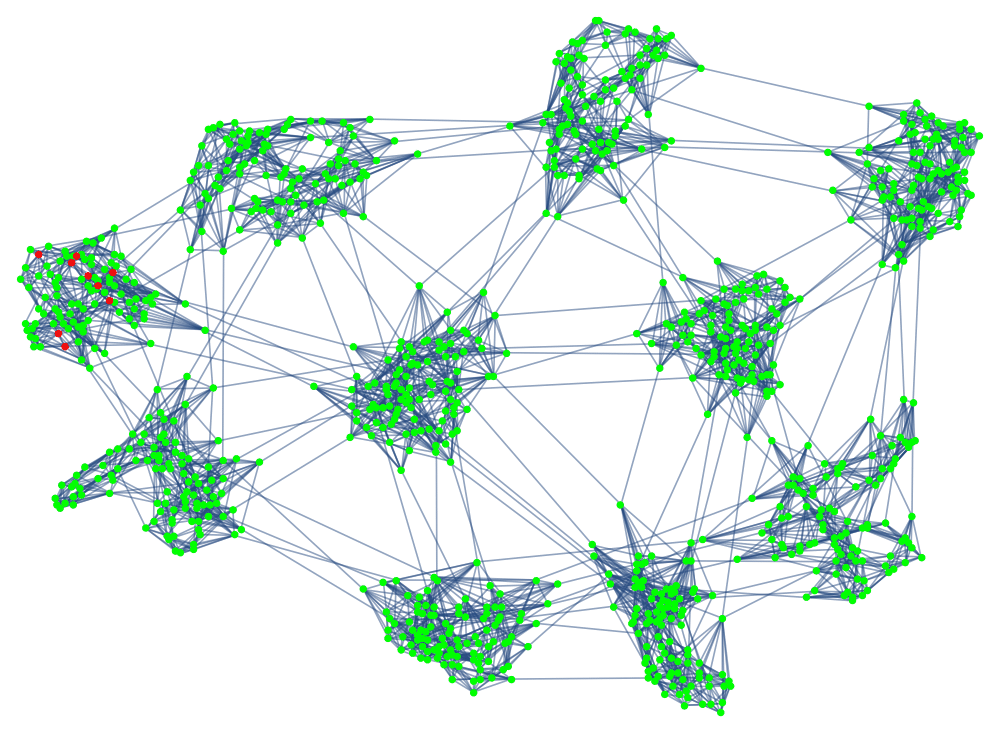
Full paper available here.
In March of 2020, at the beginning of the COVID-19 pandemic in the US, there was endless talk of disease spread modeling. ODE SIR models were popular, but they were often superseded by other models because of their assumptions of uniform mixing, and the difficulty of modeling the (undoubtedly important) geographic aspects of an global pandemic.
This lead to the rise of what I'll call "bouncing ball models", best exemplified by this Washington Post article which was popular at the time. These models were fun, and it was easy to model and visualize the geographic aspect of a pandemic.
However, it bothered me that there were so many open assumptions with all of the most popular models. Why should we think that the human contact network is anything like these bouncing balls (which behave like particles in a gas)? In ODE SIR models, why should we assume uniform mixing? More generally, why should we assume that the distribution of recovery times is normal (as some models did), or that degree distribution of the contact network is binomial (as others did)? All of these models made assumptions of this kind, but rarely (if ever) did the modelers 1) make their assumptions explicit, and 2) measure the sensitivity of their results to their assumptions, leading to situations where it is unclear to what extent the behavior of the model is a consequence of modeling assumptions with no basis in reality.
I decided to develop a very flexible version of a network SIR model, and by exploring the parameter space I could try to find results that are robust to changes in the modeling assumptions. This was especially important in the early days of the pandemic when most of the real modeling parameters were unknown.
I started by making a post on the Wolfram Community. After that got some traction, an epidemiologist friend of mine recommended that it be cleaned up and published in the Complex Systems journal, which is probably the best place to read it.
If I were to recreate this project today, I would probably do some things differently, but I think too many models are developed without experimenting to measure their sensitivity to arbitrary modeling assumptions.